
Each year the aci symposium hosts leaders in multimodal learning to share ideas and proJects.
SIGN UP TO LEARN MORE
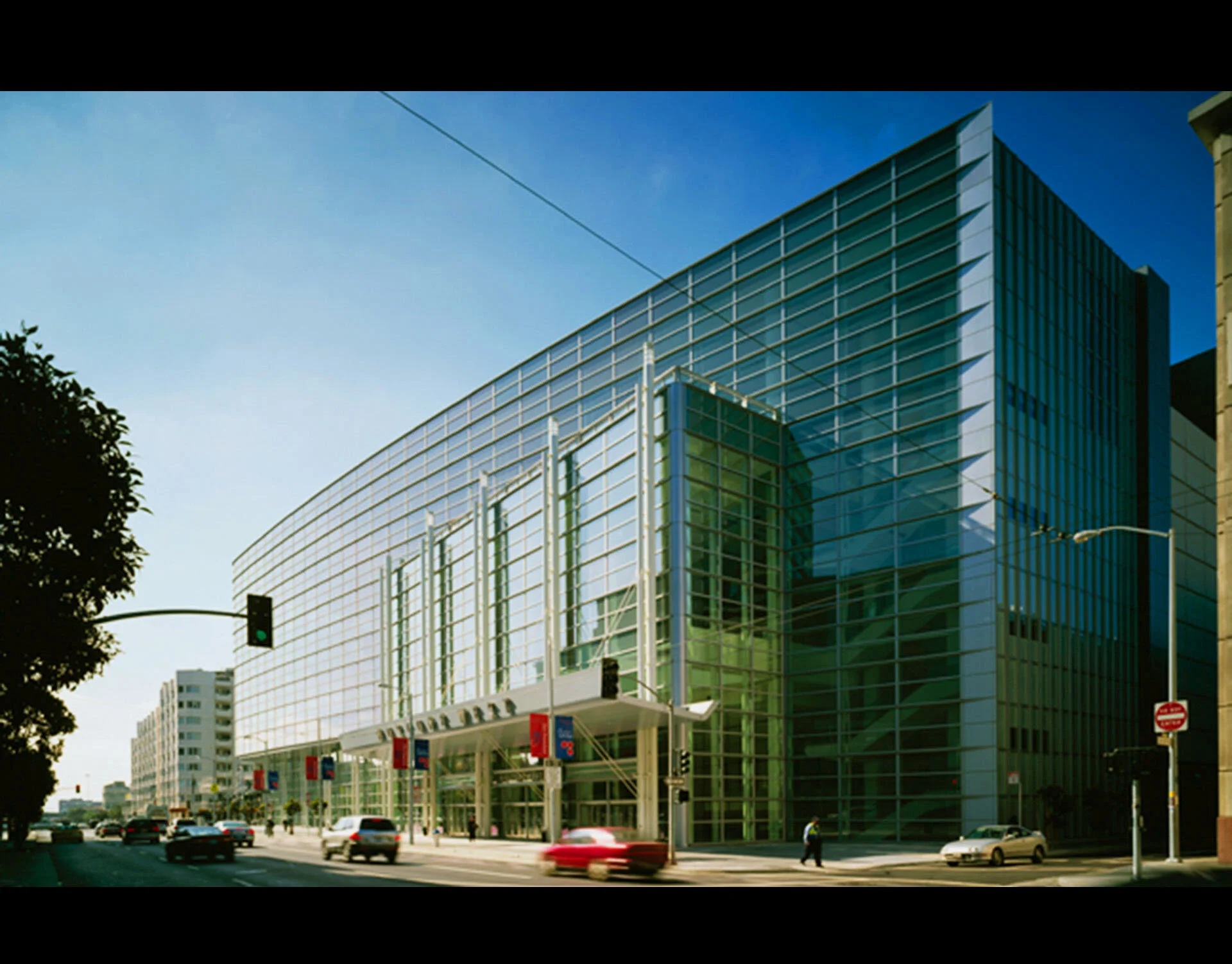
Articles and videos on multimodal learning
Lukasz Kaiser on virtual beings and where to find them
At the Virtual Beings Summit, Lukasz discusses how virtual beings have been in the field of understanding text. He shares how he's trying to mix different modalities to make virtual beings more interactive.
Google AI chief Jeff Dean interview: Machine learning trends in 2020
On Venture Beat, “Google AI chief Jeff Dean, gives talks at workshops about how machine learning can help confront the threat posed by climate change and how machine learning is reshaping systems and semiconductors.”
Top minds in machine learning predict where AI is going in 2020
On Venture Beat, their journalists “turned to some of the keenest minds in AI to revisit progress made in 2019 and look ahead to how machine learning will mature in 2020.”
Multimodal learning: The future of artificial intelligence
Currently, AI devices work independently of one another, with high volumes of data flowing through each device. As AI continues developing, these devices will be able to powerfully work in accordance with one another, unveiling the full potential of AI.
Multimodal learning is in right now — here’s why that’s a good thing
“Classification, decision-making, and HMI systems are going to play a significant role in driving adoption of multimodal learning, providing a catalyst to refine and standardize some of the technical approaches,” said ABI Research chief research officer Stuart Carlaw in a statement. “There is impressive momentum driving multimodal applications into devices.”
Multimodal AI: Computer Perception and Facial Recognition
Today, machines are closer than ever to replicating human perception of the external world. The catch? Mainstream machine learning or machine perception is more closely related to a human dream.
Neural-Network Can Identify a Melody Through Musicians' Body Movements
Music is both an auditory and visual experience. When watching an ensemble of musicians, we take visual cues to aid us to differentiate who is playing what.
Researchers Are Now Giving Neural Networks Virtual Drugs
A team of researchers has come up with a new way to test psychedelic drugs that does not require any human participation, as reported by PsyPost. They plan on administering virtual drugs to neural networks and studying their effects.
What is multimodal AI?
Multimodal AI isn’t new, but you’ll start hearing the phrase more outside core deep learning development groups. So what is multimodal AI, and why is it being called ‘the future of AI’?
The Endless Opportunities and Few Challenges of Multimodal AI
Billions of petabytes of data move through AI devices consistently. Nonetheless, at this moment, the vast majority of these AI devices are working independently of one another.
Facebook’s New AI Teaches Itself to See With Less Human Help
Now, Facebook has shown how some AI algorithms can learn to do useful work with far less human help. The company built an algorithm that learned to recognize objects in images with little help from labels.
Fruit Fly Brain Hacked For Language Processing
This team has hacked the fruit fly brain network to perform other tasks, such as natural language processing. It's the first time a naturally occurring network has been commandeered in this way.
Neuroscientists find a way to make object-recognition models perform better
MIT neuroscientists have developed a way to overcome computer vision models’ vulnerability to “adversarial attacks,” by adding to these models a new layer that is designed to mimic V1, the earliest stage of the brain’s visual processing system.
Toward a machine learning model that can reason about everyday actions
A computer vision model developed by researchers at MIT, IBM, and Columbia University can compare and contrast dynamic events captured on video to tease out the high-level concepts connecting them.
AI Needs To Learn Multi-Intent For Computers To Show Empathy
This will be the move from AI being able to interpret intent, to it being able to comprehend multi-intent… and so be able to infer deeper levels of contextual purpose from any given human (or indeed machine) generated statement or action.
AI armed with multiple senses could gain more flexible intelligence
Human intelligence emerges from our combination of senses and language abilities. Maybe the same is true for artificial intelligence.
The importance of forgetting in artificial and animal intelligence
The surprising dynamics related to learning that are common to artificial and biological systems.
Towards the end of deep learning and the beginning of AGI
How recent neuroscience research points the way towards defeating adversarial examples and achieving a more resilient, consistent and flexible form of artificial intelligence
Important papers in history of multimodal learning
Google Research: Looking Back at 2020, and Forward to 2021
“The goal of Google Research is to work on long-term, ambitious problems across a wide range of important topics — from predicting the spread of COVID-19, to designing algorithms, to learning to translate more and more languages automatically, to mitigating bias in ML models. In the spirit of our annual reviews for 2019, 2018, and more narrowly focused reviews of some work in 2017 and 2016, this post covers key Google Research highlights from this unusual year. For a more comprehensive look, please see our >800 research publications in 2020. This is a long post, but is grouped into many different sections, which you can jump to directly using the table below. Hopefully, there’s something interesting in here for everyone!”
One model to learn them all
“Abstract - Deep learning yields great results across many fields, from speech recognition, image classification, to translation. But for each problem, getting a deep model to work well involves research into the architecture and a long period of tuning. We present a single model that yields good results on a number of problems spanning multiple domains. In particular, this single model is trained concurrently on ImageNet, multiple translation tasks, image captioning (COCO dataset), a speech recognition corpus, and an English parsing task. Our model architecture incorporates building blocks from multiple domains. It contains convolutional layers, an attention mechanism, and sparsely-gated layers. Each of these computational blocks is crucial for a subset of the tasks we train on. Interestingly, even if a block is not crucial for a task, we observe that adding it never hurts performance and in most cases improves it on all tasks. We also show that tasks with less data benefit largely from joint training with other tasks, while performance on large tasks degrades only slightly if at all.”
A Case Study of Deep Learning Based Multi-Modal Methods for Predicting the Age-Suitability Rating of Movie Trailers
In this work, we explore different approaches to combine modalities for the problem of automated age-suitability rating of movie trailers. First, we introduce a new dataset containing videos of movie trailers in English downloaded from IMDB and YouTube, along with their corresponding age-suitability rating labels. Secondly, we propose a multi-modal deep learning pipeline addressing the movie trailer age suitability rating problem. This is the first attempt to combine video, audio, and speech information for this problem, and our experimental results show that multi-modal approaches significantly outperform the best mono and bimodal models in this task.
Multimodal Learning And The Future Of Artificial Intelligence
Billions of petabytes of data flow through AI devices every day. However, right now, most of these AI devices are working independently of one another. Yet, as the volume of data flowing through these devices increases in the coming years, technology companies and implementers will need to figure out a way for all of them to learn, think, and work together in order to truly take advantage of the potential that AI can deliver.
Multimodal Machine Learning: Integrating Language, Vision and Speech
Multimodal machine learning is a vibrant multi-disciplinary research field which addresses some of the original goals of artificial intelligence by integrating and modeling multiple communicative modalities, including linguistic, acoustic and visual messages. With the initial research on audio-visual speech recognition and more recently with image and video captioning projects, this research field brings some unique challenges for multimodal researchers given the heterogeneity of the data and the contingency often found between modalities.
Multimodal Intelligence: Representation Learning, Information Fusion, and Applications
Deep learning methods have revolutionized speech recognition, image recognition, and natural language processing since 2010. Each of these tasks involves a single modality in their input signals. However, many applications in the artificial intelligence field involve multiple modalities. Therefore, it is of broad interest to study the more difficult and complex problem of modeling and learning across multiple modalities.
Jointly Fine-Tuning “BERT-like” Self Supervised Models to Improve Multimodal Speech Emotion Recognition
Multimodal emotion recognition from speech is an important area in affective computing. Fusing multiple data modalities and learning representations with limited amounts of labeled data is a challenging task.
Use of multimodality imaging and artificial intelligence for diagnosis and prognosis of early stages of Alzheimer's disease
This article is a focused review of existing research in the recent decade that used statistical machine learning and artificial intelligence methods to perform quantitative analysis of multimodality image data for diagnosis and prognosis of AD at the MCI or preclinical stages.
Time-Travel Rephotography
Many historical people are captured only in old, faded, black and white photos, that have been distorted by the limitations of early cameras and the passage of time. This paper simulates traveling back in time with a modern camera to rephotograph famous subjects. Unlike conventional image restoration filters which apply independent operations like denoising, colorization, and superresolution, we leverage the StyleGAN2 framework to project old photos into the space of modern high-resolution photos, achieving all of these effects in a unified framework. A unique challenge with this approach is capturing the identity and pose of the photo's subject and not the many artifacts in low-quality antique photos. Our comparisons to current state-of-the-art restoration filters show significant improvements and compelling results for a variety of important historical people.
Technology Validation: Sparsity Enables 50x Performance Acceleration in Deep Learning Networks
This paper demonstrates the application of Numenta’s brain-inspired, sparse algorithms to machine learning. We used these algorithms on Xilinx™ FPGAs (Field Programmable Gate Array) and the Google Speech Commands (GSC) dataset to show the benefits of leveraging sparsity in order to scale deep learning models. Our results show that sparse networks are 50 times faster than non-sparse networks on an inference task with competitive accuracy.
AdaShare: Learning What To Share For Efficient Deep Multi-Task Learning
AdaShare is a novel and differentiable approach for efficient multi-task learning that learns the feature sharing pattern to achieve the best recognition accuracy, while restricting the memory footprint as much as possible. Our main idea is to learn the sharing pattern through a task-specific policy that selectively chooses which layers to execute for a given task in the multi-task network. In other words, we aim to obtain a single network for multi-task learning that supports separate execution paths for different tasks.
Self-supervised Moving Vehicle Tracking with Stereo Sound
Humans are able to localize objects in the environment using both visual and auditory cues, integrating information from multiple modalities into a common reference frame. We introduce a system that can leverage unlabeled audio-visual data to learn to localize objects (moving vehicles) in a visual reference frame, purely using stereo sound at inference time. Since it is labor-intensive to manually annotate the correspondences between audio and object bounding boxes, we achieve this goal by using the co-occurrence of visual and audio streams in unlabeled videos as a form of self-supervision, without resorting to the collection of ground-truth annotations. In particular, we propose a framework that consists of a vision “teacher” network and a stereo-sound “student” network. During training, knowledge embodied in a well-established visual vehicle detection model is transferred to the audio domain using unlabeled videos as a bridge. At test time, the stereo-sound student network can work independently to perform object localization using just stereo audio and camera meta-data, without any visual input. Experimental results on a newly collected Auditory Vehicle Tracking dataset verify that our proposed approach outperforms several baseline approaches. We also demonstrate that our cross-modal auditory localization approach can assist in the visual localization of moving vehicles under poor lighting conditions.
The sound of motions
Sounds originate from object motions and vibrations of surrounding air. Inspired by the fact that humans is capable of interpreting sound sources from how objects move visually, we propose a novel system that explicitly captures such motion cues for the task of sound localization and separation. Our system is composed of an end-to-end learnable model called Deep Dense Trajectory (DDT), and a curriculum learning scheme. It exploits the inherent coherence of audio-visual signals from a large quantities of unlabeled videos. Quantitative and qualitative evaluations show that comparing to previous models that rely on visual appearance cues, our motion based system improves performance in separating musical instrument sounds. Furthermore, it separates sound components from duets of the same category of instruments, a challenging problem that has not been addressed before.
New tricks from old dogs: multi-source transfer learning
This is multi-source transfer learning: applying the knowledge gained from multiple domain sources. Pre-trained neural networks are everywhere these days, but each tends to have a very narrow view of the world, not unlike your aunt or uncle. Taken individually, these “old dog” networks are often quite brittle and unhelpful. Taken together, though, they can teach us quite a lot.